Using data to boost your business growth
Introduction
Using data and artificial intelligence (AI) has become a key strategic leverage point for anyone looking to grow their business. When harnessed to the power of AI, data can open up doors to opportunities that were previously unimaginable, optimising processes, personalising client experiences, and anticipating market trends.
In this article, we’ll take a look at how businesses can use these technologies to drive their growth, focusing on data collection and management, advanced analysis, such as through machine learning (AI algorithms), and the implementation of data-driven strategies.
- Data collection and management in 3 simple steps
A company’s capacity to collect, manage and use its data is vital to its growth. Business data, both internal and external, provides valuable information that can be used to enhance decision-making, optimise operations, and create competitive advantages.
- Identifying the right data sources
Once you’ve put your finger on the issue, the first step to using data effectively consists of identifying the right data sources.
By “right”, we mean the relevant data sources that will respond to a specific question and/or address a particular usage case upstream (because it all starts with usages). These relevant data sources also need to provide high-quality data that can be used without creating any sense of bias or skewing results (due to insufficient completion, etc.).
In terms of the data itself, there are two kinds: internal data and external data.
Internal data comes from within your company and includes information on sales, prospects, clients, employees, suppliers, and overall performances.
For example, this data could be sourced from a customer relationship management (CRM) system, which logs interactions with clients, offering insights into their behaviours and preferences. Your enterprise resource planning (ERP) systems can also be a valuable data source, as they collect information on internal processes such as stock management and production. As for your Information System Departments, their ticketing systems record IT support data that can give you insights into how their tools are performing.
When it comes to external data, you’ll get it from open data, market data, information on competitors and sector trends. This data provides you with valuable information that can then be incorporated into your internal data for a more comprehensive overview.
- Setting up data collection systems
Once you’ve identified the right data sources, it’s absolutely essential that you then determine the right tools to use for data collection.
CRM systems can log and organise client interactions, whilst ERP systems pool data from various departments to give you a centralised management protocol.
IoT (Internet of Things) sensors are also used to collect data in real time. For example, in the manufacturing sector, IoT sensors monitor machines and equipment to provide a continuous stream of data on their performances. Using this data, manufacturers can get ahead of any breakdowns or failures and avoid them with preventative maintenance.
- Data management and storage
After collecting internal data, you need a dedicated and secure environment that complies with the applicable regulations, where you can test various models and analyse the data efficiently. This process also allows you to centralise any external data. These environments can either be hosted on-site (on your premises) or on the cloud.
There’s a lot more to data management than just figuring out how you’ll collect it. Cloud storage solutions and databases offer flexible and scalable options, enabling you to store big volumes of data. Relational database management system (RDBMS) software and NoSQL databases are often the solutions of choice for various requirements with structured and unstructured data.
Then, there’s one of the most critical aspects to consider: data security. With cyberattacks and data breaches on the rise, having the appropriate security measures in place to protect their sensitive information is now a firm non-negotiable. This includes ensuring compliance with the applicable regulations, such as the European General Data Protection Regulation (GDPR).
It’s all well and good having efficient data collection processes in place, but they’re not worth anything if they’re not analysed and exploited correctly.
- Data analysis and machine learning
Analysing data and applying machine learning are key elements in the transformation of raw data into exploitable information and competitive advantages. By using advanced modelling techniques, businesses can extract valuable insights that will help them to make good strategic decisions and optimise their operations.
Transforming data into information that you can use
The data analysis process starts with preparing the data so that it can be used effectively.
This is a key step that’s not to be neglected, as raw data is often disorganised and incoherent. Data cleaning involves getting rid of any duplicates, correcting any mistakes, and managing any missing values. This ensures what we refer to as “data quality”. Once this step is complete, the data can be transformed into indicators over various time frames: it’s ready for analysis.
There are different data analysis techniques to cater to the company’s specific needs:
- Descriptive analyses provide an insight into previous performances and events;
- Predictive analyses use statistic models to anticipate future trends;
- Prescriptive analyses suggest optimal actions based on predictions.
Applications for machine learning (AI algorithms)
Machine learning plays a major role in advanced data analysis.
Machine learning algorithms learn from the data they’re provided with and can generate predictions or classifications without being explicitly programmed for each task. This means that companies can detect models within their data and automatise complex processes.
Predictive models are often used to forecast sales, anticipate stock requirements, and identify market trends. For example, a machine learning model can analyse previous sales data and economic data to predict future demand for products. This can then facilitate day-to-day management and speed up processes. By basing processes and decisions on reliable data, the risks of errors are very low.
For marketing, client segmentation algorithms enable the client base to be split into consistent groups, facilitating targeted marketing campaigns.
These techniques can also be used to limit repetitive tasks for employees and boost operational efficiency (e.g., by automatically reading documents to directly convert the written information in the document into a database without need for manual input).
Analysis platforms and tools
To capitalise on data analyses and machine learning, companies can use various tools and data aggregation platforms to take their data to the next level.
Business intelligence solutions, like Tableau and Power BI, allow users to access an interactive vision of their data, facilitating insight communication and exploration. These tools incorporate advanced analysis features that can be used to create dynamic dashboards and detailed reports. These are great choices that allow for information to be shared optimally with operating and strategic teams.
Machine learning platforms, like TensorFlow, scikit-learn and Microsoft Azure Machine Learning offer powerful environments for developing, training and deploying models. These platforms provide users with preset function and algorithm libraries, along with tools for evaluating and optimising models.
In addition, companies can use cloud-based services to enhance their processing capacity and store huge volumes of data. Cloud providers, such as AWS, Google Cloud and Microsoft Azure, offer flexible storage and calculation services that cater to business’ growing needs.
Data analysis and machine learning are essential elements when it comes to unlocking and capitalising on the full potential of business data. By transforming data into exploitable information and through the application of machine learning techniques, companies can make more informed decisions, anticipate future trends and automatise critical processes, all whilst also reducing the likelihood of errors to almost zero. This ability to analyse and interpret data is crucial if you want to launch strategies built on data and AI.
III. Implementing strategies based on data and AI
So, we’ve gone through how you can better collect and organise your data. Now, let’s look at how you can make the most of it: by implementing the right strategies.
Developing data-driven strategies
The first step towards incorporating data and AI consists of aligning the data collected by the company with the company’s objectives.
For example, a company looking to boost client satisfaction may focus on analysing client feedback data to identify areas for improvement.
Once the objectives have been set, it’s essential to put together detailed action plans that use the insights derived from the data.
This includes defining key performance indicators (KPIs) to measure the progress and efficiency of actions taken.
Measuring impacts and making adjustments
The implementation of strategies based on data and AI needs to be followed up with a continuous evaluation of their impacts.
Companies need to set up performance tracking systems that will monitor the KPIs determined during the planning phase. Interactive dashboards and analytical reports play a key role in this step, providing an overview of detailed insights and the performances of each action.
Evaluating performances will not only allow you to measure the impact of adjustments made to the company’s business strategy but will also allow you to identify areas that need further adjustments.
For example, if a marketing campaign doesn’t generate the results you were expecting, a data analysis can reveal specific issues, such as inefficient targeting or the wrong message. Companies can then adjust their strategies respectively, using the new data to refine predictive models or personalisation algorithms.
Lastly, AI means that you can always make extra use of the data collected by the company and it ensures that each change generates its own set of new data and potentially added-value information. This new data can be used to further improve business performances. In the long-term, the company will have also made valuable time-savings by including AI management and data amongst its technical expertise.
And for the others, Apside allows them to unlock the power of their data and start to use it to its full potential. Our teams are made up of top data analysts, AI professionals (data engineers, data scientists) and architects, enabling you to go above and beyond with your strategies, building them on concrete data.
Conclusions
The use of data and artificial intelligence is no longer optional for business growth – it’s now a must-have for companies.
By collecting and efficiently managing data, using advanced machine learning techniques to analyse this data, and developing data-driven strategies, companies can transform their information into a powerful, strategic asset.
This approach not only allows companies to optimise operational aspects and personalise their marketing, but also to make more informed decisions and keep a competitive edge on the market.
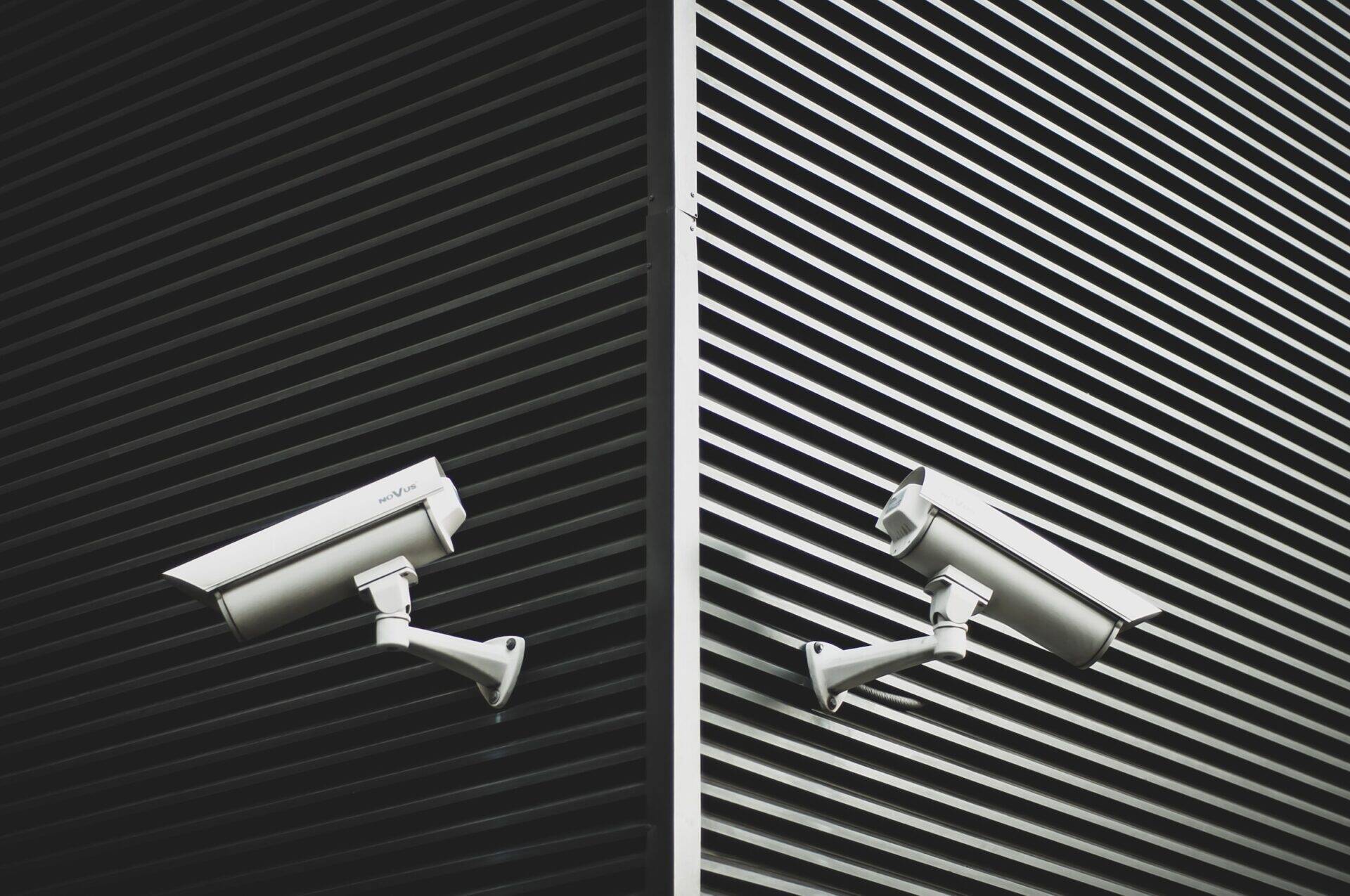
EXPERTISE CYBER
With over 40 years’ experience in IT and industrial engineering, Apside has developed expertise in cyber security thanks to a dedicated team.