[ The RAG model - #2 ] RAG, an in-depth exploration
The models used in a RAG: An in-depth exploration
In the world of artificial intelligence (AI), text generation models have made considerable progress in recent years. Among these innovations, the RAG (Retrieval-Augmented Generator) stands out as a particularly promising model. In this article, we will explore the models used in a RAG, how they work and the challenges involved in developing them.
What models are used in a RAG?
A RAG uses a combination of templates to search and generate text. The most commonly used templates are :
- Language models: Language models are used to generate text. They can be based on neural networks or Markov models (1)
- Search models: Search models are used to retrieve relevant information from a knowledge base. They can be based on search algorithms such as similarity search or relevance search.
- Selection models: Selection models are used to select the most relevant information for the task in hand. They can be based on selection algorithms such as selection by relevance or selection by precision.
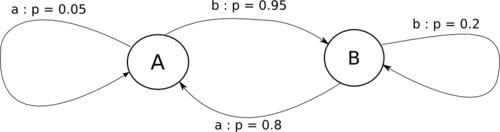
(1) Example of a Markov model
How do the models in a RAG work?
The models in a RAG work in a coordinated way to carry out the search and generate the text. Here is an overview of the process:
- Search: The search model is used to retrieve relevant information from a knowledge base.
- Selection: The selection model is used to select the most relevant information for the task in hand.
- Generation: The language model is used to generate text based on the information selected.
Advantages of models in a RAG
Templates in a RAG have several advantages over traditional text generation templates:
- Relevance: Search and selection models allow you to retrieve and select information that is relevant to the task in hand.
- Accuracy: Language models can be used to generate accurate, consistent text.
- Flexibility: Templates in a RAG can be used for a variety of tasks, such as answering questions, generating summaries or creating dialogues.
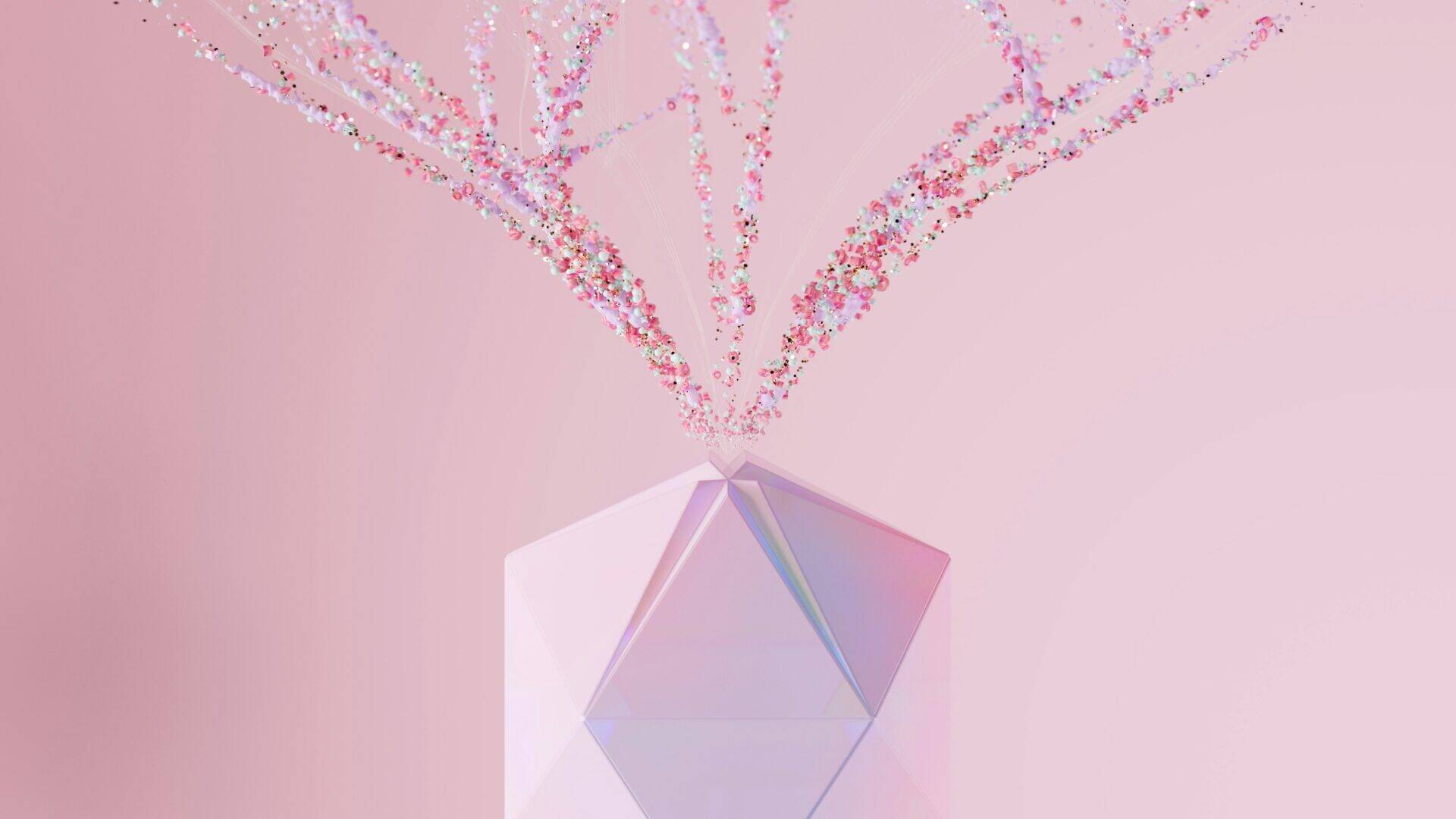
Challenges
Although the models in a RAG are promising, there are several challenges to their development:
- Quality of the knowledge base (Indexing): The quality of the knowledge base is crucial to the performance of the models in a RAG.
- Model complexity: The models in a RAG are complex and require a large amount of data and resources to function correctly.
- Performance evaluation: It is difficult to evaluate the performance of the models in a RAG, as there are no standard metrics for measuring the quality of the texts generated.
Conclusion
The templates used in a RAG are powerful tools for generating text. They enable the retrieval and selection of relevant information, and the generation of accurate and coherent text. However, there are challenges to their development, such as the quality of the knowledge base, the complexity of the models and performance evaluation. Future research should focus on improving these aspects to make GenAI models even more powerful and useful in a variety of applications.